Surrogate model-enhanced design optimization in electrical machines transforms how you approach complex design challenges. It streamlines workflows and balances competing objectives like efficiency and cost, all while improving prediction accuracy. However, you'll face challenges like ensuring model accuracy and accessing quality data. As machine complexities grow, embracing emerging machine learning algorithms can unlock new opportunities. You'll discover how these advancements can reshape electrical machine performance and efficiency in the sections that follow.
Key Takeaways
- Surrogate models streamline the optimization process in electrical machine design, enabling quicker iterations and exploration of design options.
- Challenges include maintaining accuracy while managing computational costs and ensuring data quality for effective machine learning.
- Multi-objective optimization frameworks balance competing design objectives, enhancing performance metrics like efficiency and power density.
- Future advancements in machine learning algorithms present opportunities to improve surrogate model applicability and precision in design optimization.
- Industry collaboration and continued research are essential for overcoming current challenges and achieving significant improvements in electrical machine performance and cost efficiency.
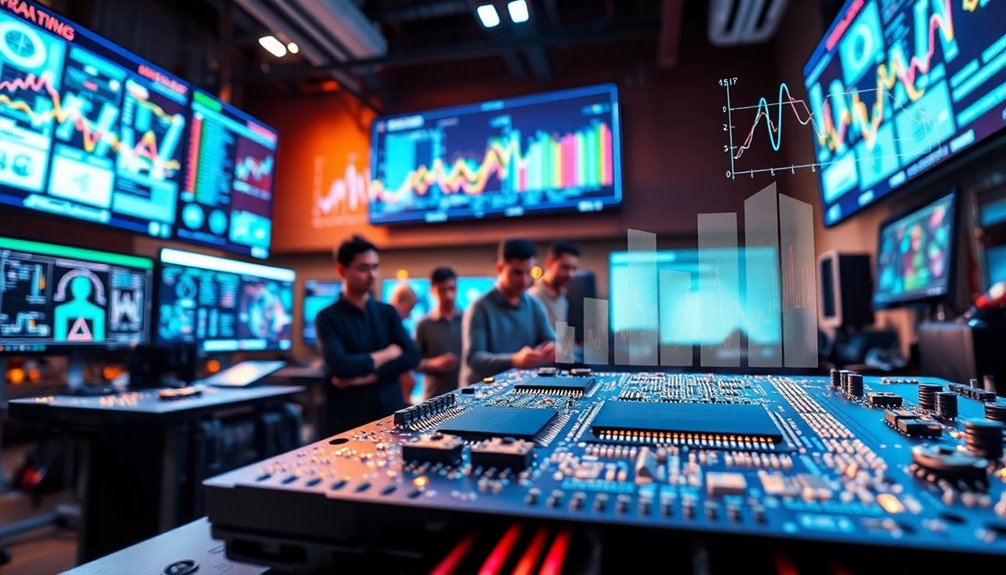
As electrical machine design grows increasingly complex, leveraging surrogate models can significantly streamline optimization processes. These models provide faster, more cost-effective solutions compared to traditional numerical simulations. You'll find that employing surrogate models can enhance your design workflow, allowing for quicker iterations and more efficient exploration of design options.
When you're considering methodologies, you can choose from physical-model, data-driven, and hybrid approaches that blend physical insights with machine learning. Each has its benefits, but remember that physical models may struggle with nonlinear phenomena, while data-driven models need extensive datasets to be effective. In this context, artificial neural networks often come into play, improving prediction accuracy and enabling you to tackle complex design challenges more effectively.
In your optimization endeavors, you might notice the significance of multi-objective frameworks. These frameworks help you balance competing design objectives such as efficiency, cost, and performance. Utilizing both analytical and machine learning methods can address the complexity of multi-objective optimization, allowing you to focus on key performance metrics like efficiency, power density, and cogging torque. By adopting a hybrid approach, you can enhance both the robustness and accuracy of your results. This approach aligns with the findings of surrogate model-assisted multi-objective design optimization as a promising technique for improving design outcomes.
However, be aware of emerging challenges. As machines grow more sophisticated, maintaining accuracy without incurring excessive computational costs becomes a pressing issue. You may also encounter challenges related to data quality and availability, which can hinder the effectiveness of machine learning-based surrogate models.
Integration with other optimization techniques, such as topology optimization, is vital for overcoming these hurdles. Industry adoption hinges on demonstrating the cost savings and performance improvements these models can offer. Advancements in machine learning algorithms present exciting opportunities to enhance the precision and applicability of surrogate models.
Frequently Asked Questions
What Are the Key Benefits of Using Surrogate Models in Design Optimization?
Surrogate models in design optimization offer you several key benefits. They significantly reduce computational costs and time, allowing you to explore a wider design space and conduct more iterations.
You'll find improved accuracy and reliability by integrating both data-driven and physics-based insights. Additionally, their flexibility lets you adapt to different scenarios and optimization algorithms, enhancing your ability to evaluate complex relationships and discover optimal solutions efficiently.
How Do Surrogate Models Compare to Traditional Optimization Methods?
You might worry that surrogate models can't match the accuracy of traditional optimization methods, but they offer significant advantages.
Surrogate models reduce computational burden, allowing you to evaluate designs much faster. While they may be slightly less accurate, advancements like Waveform-Targeted Surrogate Modeling enhance reliability.
Plus, they save costs and scale better in complex design spaces. Ultimately, embracing surrogate models can lead to more efficient and innovative design processes.
What Industries Are Adopting Surrogate Model-Enhanced Design Optimization?
You're likely to see surrogate model-enhanced design optimization being adopted in various industries, especially aerospace and electrical engineering.
Aerospace uses it for optimizing designs in aerodynamics and propulsion systems, while electrical engineering benefits from improved efficiency in machines.
Additionally, sectors focusing on reliability and thermal management are also incorporating these models to tackle complex challenges, reduce costs, and enhance performance.
This trend is paving the way for innovative solutions across multiple fields.
Are There Specific Software Tools for Implementing Surrogate Models?
If you're diving into the world of surrogate models, you've hit the jackpot!
Tools like the Surrogate Modeling Toolbox (SMT) and COMSOL Multiphysics are your trusty sidekicks.
SMT's got a treasure trove of methods, while COMSOL brings fast model evaluations to the table.
You'll also find general software leveraging machine learning, making your life easier.
With these tools, you'll conquer design optimization like a wizard casting spells!
What Skills Are Needed to Work With Surrogate Models in Engineering?
To work effectively with surrogate models in engineering, you'll need a mix of skills.
Start with a solid understanding of machine learning and statistical modeling. You should be proficient in data analysis and have programming skills in languages like Python or R.
Familiarity with design of experiments, multi-objective optimization, and validation techniques is crucial.
Don't forget the importance of clear communication and collaboration with interdisciplinary teams to ensure project success.
Conclusion
In the ever-evolving landscape of electrical machine design, embracing surrogate models is like planting seeds in fertile ground; they promise growth and innovation. As you navigate the challenges ahead, remember that each obstacle is a stepping stone, leading to richer opportunities. By harnessing these powerful tools, you're not just optimizing designs—you're sculpting the future of technology. So, take that leap, and let your creativity illuminate the path to groundbreaking advancements. The horizon is bright, and the journey is yours.